Outliers and Influential Cases
21 พฤศจิกายน 2566 - เวลาอ่าน 3 นาที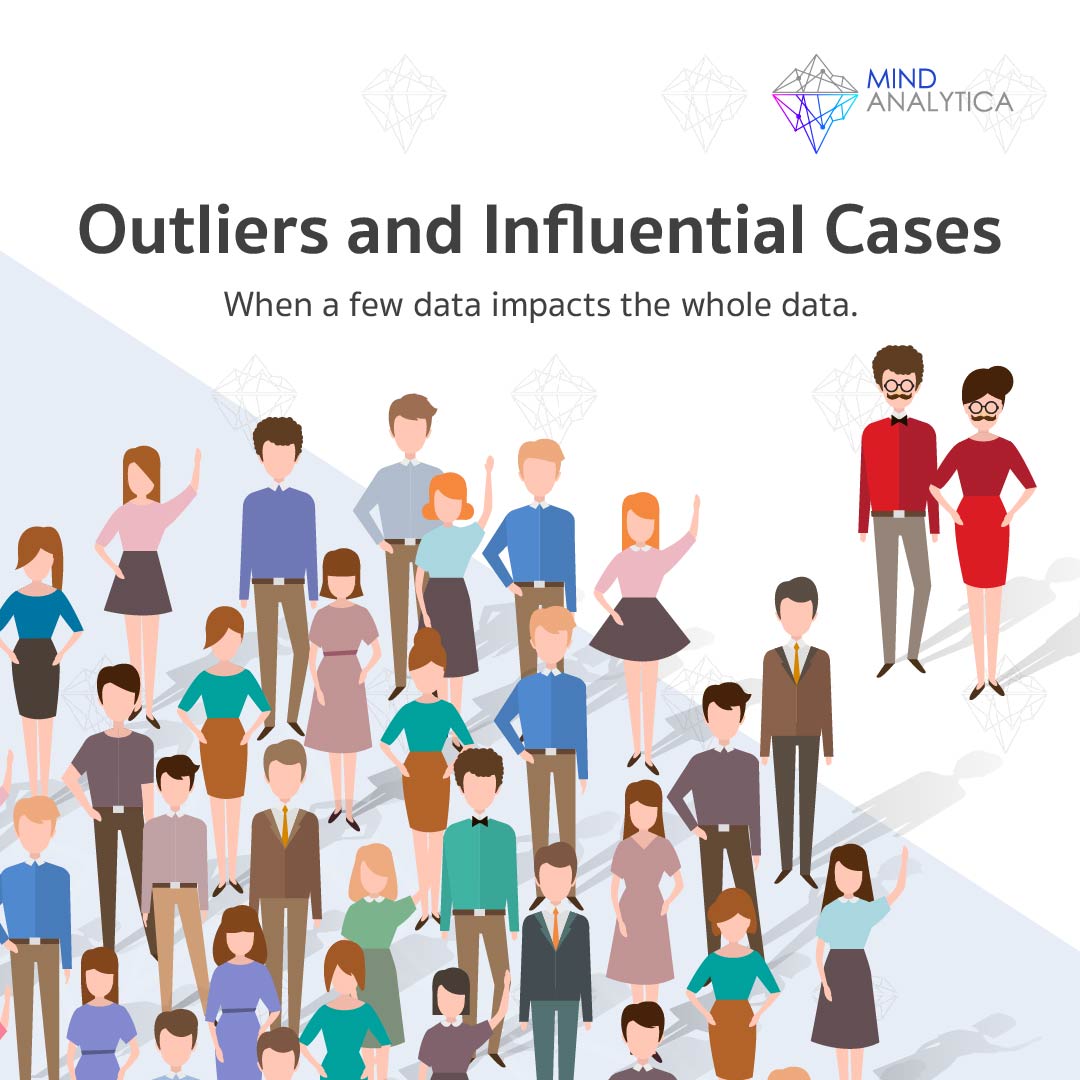
When a few data impacts the whole data.
In research studies, it's often found that within a sample group, certain data points with unexpectedly high or low scores lead to flawed data analysis, which is undesirable in research. These data points, affecting the analysis, fall into two categories: outliers and influential cases.
Outliers are data points that significantly differ from the sample group or population. For instance, in a sample group of students with grades ranging from 2.0 to 3.2, having one student with a grade of 4.0 constitutes an outlier. Moreover, outliers may also depend on more than one variable. For example, a Thai individual might have a height of 180 cm, a range typically observed among Thais. However, if this person is only 11 years old, they'd also be considered an outlier.
On the other hand, influential cases are outliers that alter the outcome of data analysis. For example, in a hypothetical case study examining the impact of psychological therapy on reducing anxiety, data was collected from a sample of 100 individuals who underwent therapy, compared to those who did not. The results showed that the therapy group had lower anxiety scores than the non-therapy group. However, upon further examination, it was observed that only 4-5 individuals in the therapy group showed significantly reduced anxiety scores, while the rest had high anxiety scores. This led to a situation where the average anxiety score of the entire therapy group was lower than expected due to the influence of these few individuals.
If an outlier doesn't change the outcome of the data analysis, it's not considered influential. Additionally, while one outlier may influence one analysis, it might not affect another. For example, a student with a perfect 4.0 GPA may influence the average GPA in a study, but when predicting university entrance exam scores, including or excluding such students in the analysis didn't change the GPA-based predictions. Hence, in this case, the outlier isn't considered influential.
When researchers encounter outliers and influential data, they should start by considering the causes that lead to the existence of these outliers. Possible causes might include data management errors, such as incorrect data entry, unintentional data modification, or failing to assign numbers for missing data. Another cause could be poor quality responses from the sample group, where respondents quickly answer questions without reading them properly.
Dealing with outliers and influential data, researchers should exclude the data of those specific individuals from the analysis to ensure the analysis reflects reality as accurately as possible. However, if outliers naturally exist within the sample group, such as students with a 4.0 GPA among others with lower scores, this is considered a natural representation. In such cases, the scores of these individuals shouldn't be excluded from the analysis but rather further analyzed separately from the group with lower scores.
Moreover, advanced statistical knowledge has suggested Finite Mixture Models, which examine whether there might be subgroups within a sample group. For instance, in analyzing the results of psychological therapy, discovering that 4-5 individuals benefited while others didn't, the mixture analysis could show two subgroups: one that benefited and another that didn't. This analysis helps to understand the effectiveness of therapy more deeply.