When Users Try to Avoid Algorithms
19 มิถุนายน 2567 - เวลาอ่าน 4 นาที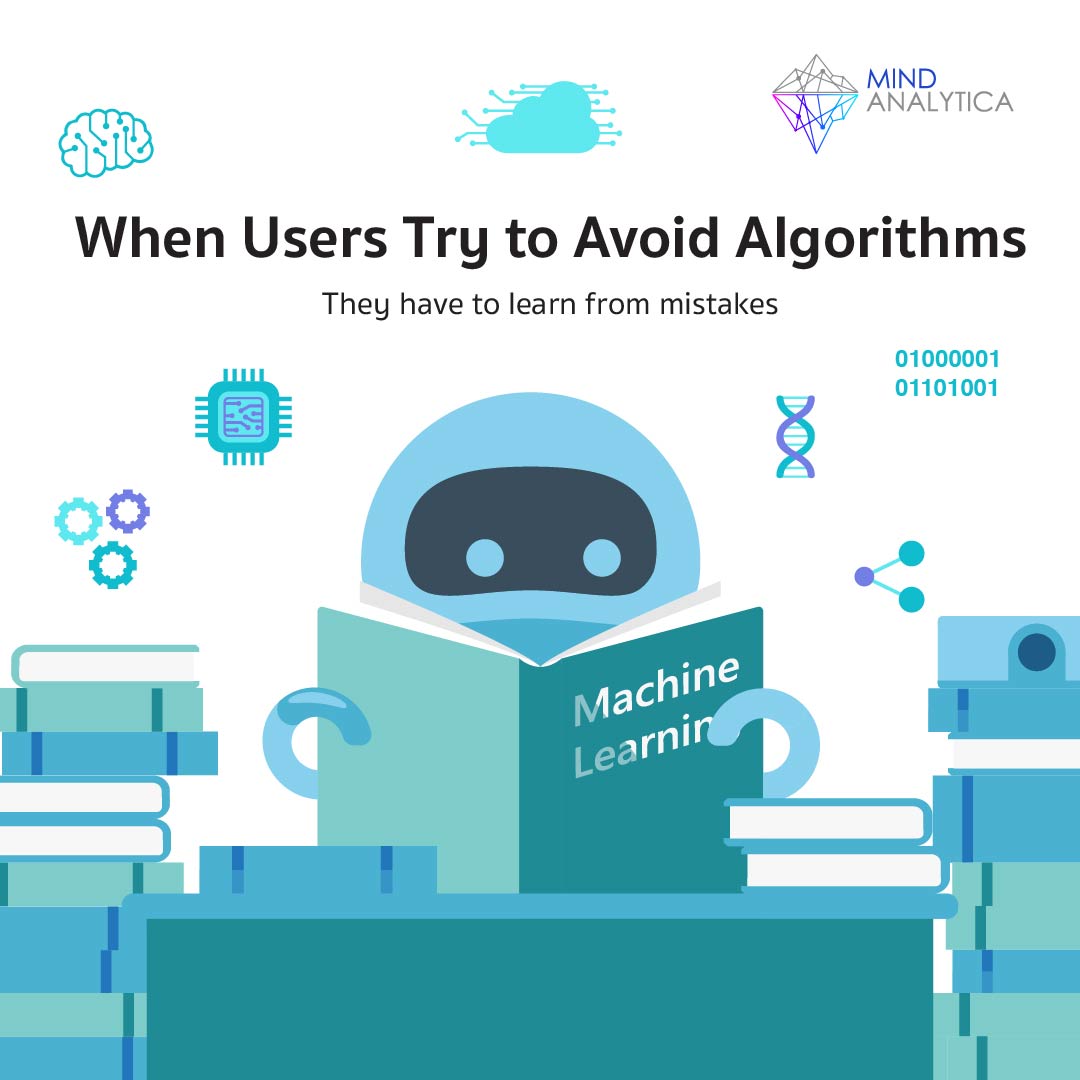
They have to learn from mistakes
Advancements in predictive algorithm technology, particularly in the consumer realm, have revolutionized how we shop online, find entertainment at home, and even seek medical advice. Machine learning and artificial intelligence models enable companies to deliver personalized products and content to consumers with greater precision and insight. This shift has not only transformed consumer behavior, interactions, and purchasing decisions but has also introduced a new dynamic: algorithm aversion.
While consumers traditionally relied on human recommendations from friends, experts, or marketers, they now encounter recommendations from algorithms. However, this shift has led to a differential treatment of recommendation sources. Despite algorithms playing an increasingly prominent role in consumer decision-making, algorithm aversion persists, with consumers favoring human recommendations over algorithmic ones, even when evidence suggests superior performance from algorithms. This preference stems from the perception that algorithms cannot capture individual nuances and are often perceived as unethical and lacking human judgment.
On the other hand, consumers who embrace algorithmic recommendations often cite contexts requiring objective, data-driven advice over subjective, sentiment-based guidance. However, errors are inevitable, regardless of whether they stem from humans or algorithms. The ability to learn from mistakes, therefore, becomes a crucial factor in algorithm development.
The Study
Researchers from Yale University conducted a six-part study to investigate how algorithm learning from mistakes affects user perception and decision-making.
Part 1: Trust in Human vs. Algorithmic Recommendations
Participants were asked to rate their trust in human and algorithmic recommendations in both concrete (e.g., medical treatment) and abstract (e.g., personality assessment) domains. Results indicated that participants perceived humans as more knowledgeable than algorithms in both domains.
Part 2: The Impact of Error Rates
Despite being informed about the error rates of both humans and algorithms, participants continued to favor human recommendations. This finding suggests that even when acknowledging equal error probabilities, participants perceive human mistakes as less detrimental than algorithmic ones.
Part 3: Demonstrating Algorithm Learning
Two groups of participants were presented with different information about an algorithm's learning progress over a year. One group saw the algorithm's accuracy improve, while the other saw only the error rates. Both groups still perceived the algorithm as less capable of learning than humans. However, the group observing the algorithm's improvement showed increased trust in the algorithm, comparable to their trust in humans.
Part 4: Decision-Making with Real-World Incentives
This experiment introduced real-world incentives to assess decision-making. Participants were presented with personality assessments from a human, a traditional algorithm, a machine learning algorithm, and a learning algorithm. When faced with potential rewards for accurate assessments, 66.3% of participants chose the learning algorithm, 33.7% chose the human, 50.5% chose the traditional algorithm, 49.5% chose the human, and 26.7% chose the traditional algorithm, 73.3% chose the human. This experiment reflects real-world decision-making scenarios, where incentives influence choices, and participants still opted for the learning algorithm over the human.
Part 5: Linguistics on Users' Perception of the Algorithm
This experiment simulated online dating by presenting participants with success rates from different matchmakers: a human, a traditional algorithm, a machine learning algorithm, and a learning algorithm. Participants rated their trust in each matchmaker. Results showed similar levels of trust in humans, machine learning algorithms, and learning algorithms, with lower trust in traditional algorithms. This suggests that using terms like "machine learning" can enhance user trust and perception, aligning with the results of Part 3.
In a separate experiment, participants were asked to choose between an algorithm and themselves to make a decision about artwork. One group saw the term "machine learning algorithm," while the other saw only "algorithm." The group exposed to "machine learning algorithm" showed a higher preference for the algorithm.
Part 6: Algorithm Performance with Low Accuracy and Slow Learning
The previous experiments focused on high-performance algorithms. This experiment investigated how participants would respond to an algorithm with low accuracy (65%) and slow learning. Even with slow learning, participants still preferred the learning algorithm over the non-learning algorithm.
Conclusion
Algorithm aversion persists despite its widespread use in consumer decision-making. However, the study's findings suggest that learning from mistakes, regardless of overall performance, can mitigate algorithm aversion. Additionally, using terms that convey learning, such as "machine learning," can enhance user trust and preference for algorithms. As businesses increasingly rely on algorithms to deliver content and product recommendations, incorporating these insights into communication strategies can build user trust and encourage algorithm adoption.
Original Article
Reich, T., Kaju, A., & Maglio, S. J. (2023). How to overcome algorithm aversion: Learning from mistakes. Journal of Consumer Psychology, 33(2), 285-302.